Prerequisite concepts
For an $n-$dimensional function $f$, the gradient of $f$ at a point is an $n-$dimensional vector
The Master Formula states that a small change in a function $df$ is the dot product of the gradient of the function with a small step $d \vec r$ through the domain of the function: \[df = \vec \nabla f \cdot d \vec r.\] In order to maximize the change in $f$ one must maximize this dot product, which happens when the small step $d \vec r$ is parallel to the gradient. Or, turning this statement around, the gradient points in the direction in which the function is increasing the most.
Acting out the Gradient

Oftentimes, students are not correctly interpreting situations geometrically when it seems they are. This activity is designed to test the ability of a class to represent the gradient physically for a function $f(x,y)$ that represents an elliptical hill. In the activity, the students, distributed throughout the classroom, represent a vector field at various points. Students are asked to point, using their right arm, in the direction of the gradient. The instructor will also have the opportunity to clarify the proper gradient direction (in the $xy$-plane) for students that are pointing incorrectly ("up" the hill).
Representations used
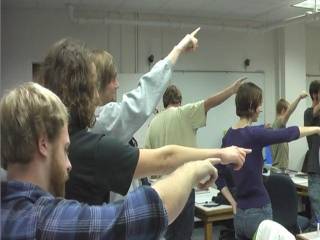
Concepts taught
The gradient of an $n$ dimensional function is $n$ dimensional. This is not to be confused with the gradient having the same physical dimensions (e.g., Newtons) as the original function.
The magnitude and direction of the gradient depends on the behavior of the function infinitesimally near the point of interest. One implication of this is that the gradient does not point in the direction of maxima a finite distance away (except sometimes accidentally), but rather in the direction of steepest slope at the point of interest.